Colin here. We read a lot about the various applications of artificial intelligence and machine learning. Sometimes a bit too much, if you believe the dystopian narratives that seem to flood social media and the press. So I thought this piece from The Verge, sent via our pal Benjamin Palmer, was both human and refreshing. AI is being used to help old video games look new. The graphics and resolutions are being improved by an interesting process:
The technique being used is known as “AI upscaling.” In essence, you feed an algorithm a low-resolution image, and, based on training data it’s seen, it spits out a version that looks the same but has more pixels in it. Upscaling, as a general technique, has been around for a long time, but the use of AI has drastically improved the speed and quality of results.
Why is this interesting?
It just seems amazing to me that people are using the techniques of art restoration and applying them to slightly dusty video games. There’s a real humanness to the whole endeavor:
It’s a job for skilled craftspeople, requiring patience and knowledge. Not every game is a good fit for upscaling, and not every upscaling algorithm produces similar results. Modders have to pick the right tool for the job before putting in hundreds of hours of work to polish the final results. It’s a labor of love, not a quick fix.
Much like Wikipedia’s moderators see themselves as the guardians of a collective body of knowledge, this trend has a lot of passionate restoration experts looking after classic games. If all of this sounds a bit abstract, here’s a screenshot:
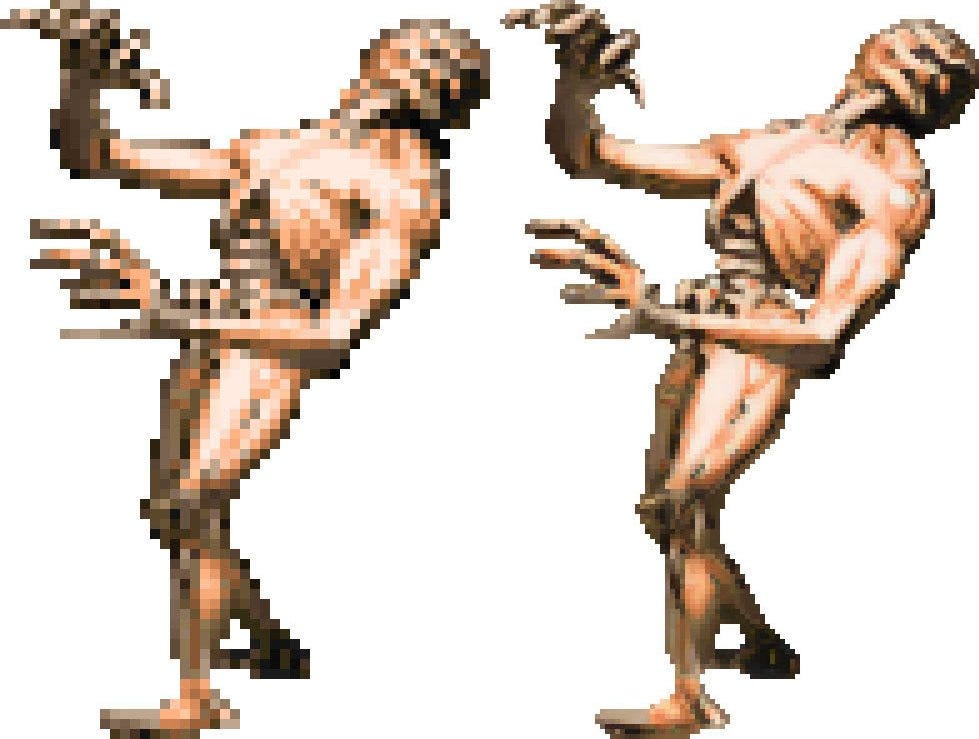
While at first the final effect might seem underwhelming, remember that this isn’t just a bunch of people touching up games by hands: it’s literally algorithms talking to other algorithms to figure out optimal ways to fill in the spaces between pixels as you expand an image. “One part of the algorithm tries to re-create this transition from low-res to high-res, while another part compares its work against the training data, seeing if it can spot the difference and rejecting the output if not. This feedback loop is how GANs improve over time.” Picasso meets Pixar. (CJN)
Chart of the Day:
A blast from the internet past: the pop vs. soda map. (NRB)
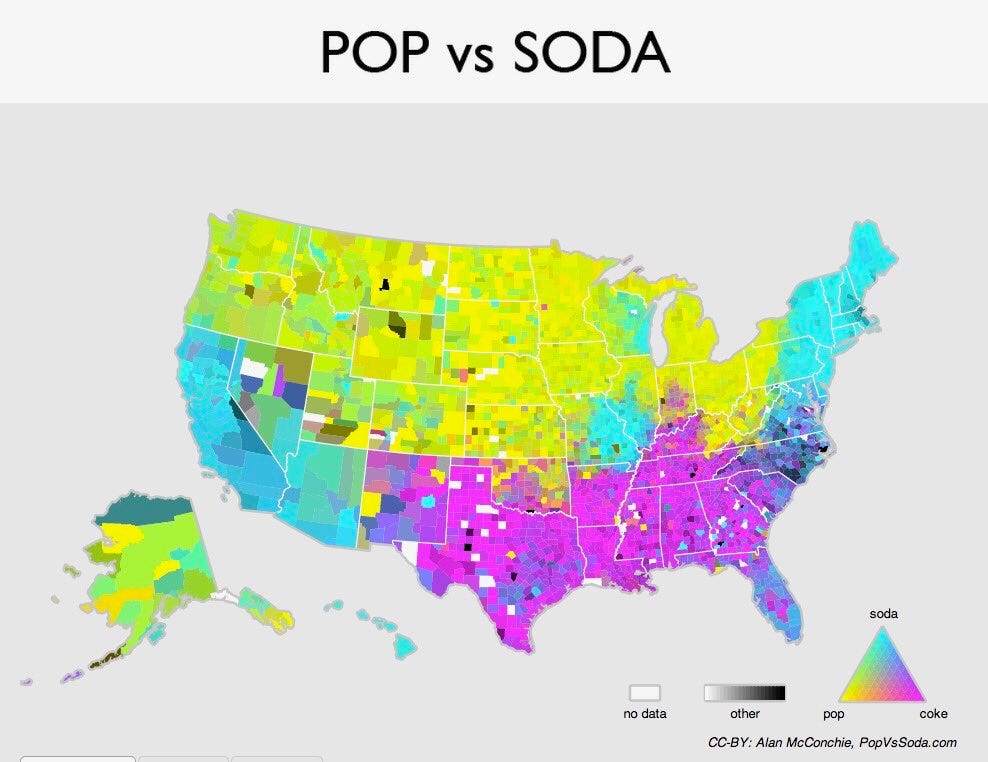
Quick Links:
WITI reader Brady wrote in with another triangle after yesterday’s edition: “The standard platoon-size (40 or so light infantry) patrol base is laid out in a triangle, with one squad (9 men) having a side, and your crew-served machine guns at each apex. I’ve always thought it was an improvement on the British Infantry square, but may be more a function of a platoon having 3 standard squads each.” Keep the links coming! (NRB)
The perils of a truly great Jeopardy! player. (CJN)
Felix Salmon mentioned one of the more interesting startup perks I’ve ever heard about: “Zoom will reimburse you for any book you buy for yourself or your family members.” (NRB)
A bunch of interesting sleep tidbits in this CNN wrap up of a sleep study recently published in the journal Sleep Health. Adults need 7-10 hours, regardless of what you may have heard, and don’t just lay in bed if you can’t sleep. “In reality, Robbins said, it takes a healthy sleeper about 15 minutes to fall asleep. If you're tossing and turning much longer than that, you should get out of bed, change the environment and do something mindless.” (NRB)
Thanks for reading,
Noah (NRB) & Colin (CJN)